Exploring Artificial Intelligence Generative Models and Their Detection Using AI Detectors
In the realm of artificial intelligence (AI), generative models stand out as fascinating and powerful tools capable of creating realistic and diverse data samples.
These models have found applications in various fields, including image synthesis, text generation, music composition, and more. However, as with any technology, there are concerns about their potential misuse, particularly in generating deceptive or harmful content. This has led to the development of AI detectors, specialized tools designed to identify such generated content and mitigate its negative impacts.
In this article, we delve into the world of generative models, explore their capabilities, and discuss the importance of AI detectors in safeguarding against misuse.
Chapters
Understanding Generative Models
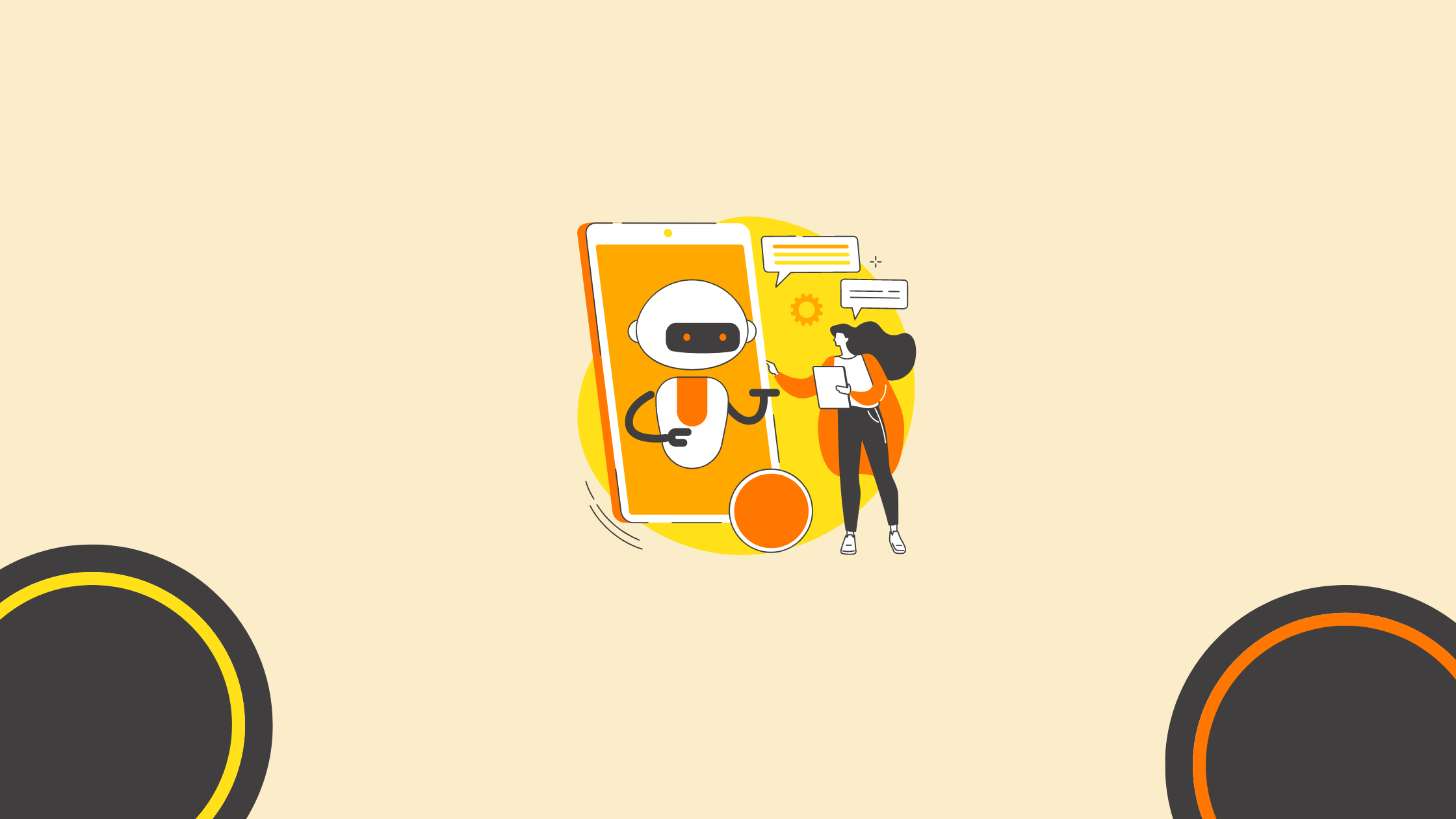
Generative models are a class of AI algorithms that learn to generate data resembling a training dataset. Unlike discriminative models that classify input data into predefined categories, generative models aim to understand the underlying structure of the data and produce new samples that are statistically similar to the training examples.
One of the most popular types of generative models is the generative adversarial network (GAN), proposed by Ian Goodfellow and his colleagues in 2014. In a GAN, two neural networks, the generator and the discriminator, are pitted against each other in a game-like scenario. The generator attempts to create realistic samples, while the discriminator tries to distinguish between real and generated data. Through this adversarial process, both networks improve iteratively, with the generator eventually learning to produce highly convincing outputs.
Other notable generative models include Variational Autoencoders (VAEs), Autoregressive Models, and Transformers. Each of these approaches has its unique strengths and weaknesses, making them suitable for different types of data generation tasks.
Potential Misuse and Risks
While generative models offer numerous benefits, there are concerns regarding their potential misuse. One of the primary worries is the generation of fake content, such as realistic images of non-existent people, forged documents, or misleading news articles. Such content could be used for malicious purposes, including spreading disinformation, impersonation, or creating fraudulent materials.
Moreover, generative models could also be employed to circumvent security measures, such as generating realistic but synthetic biometric data for identity theft or creating sophisticated phishing scams. Additionally, in sensitive domains like healthcare or finance, the generation of synthetic data resembling real patient records or financial transactions could pose significant privacy risks if not properly handled.
Introducing AI Detectors
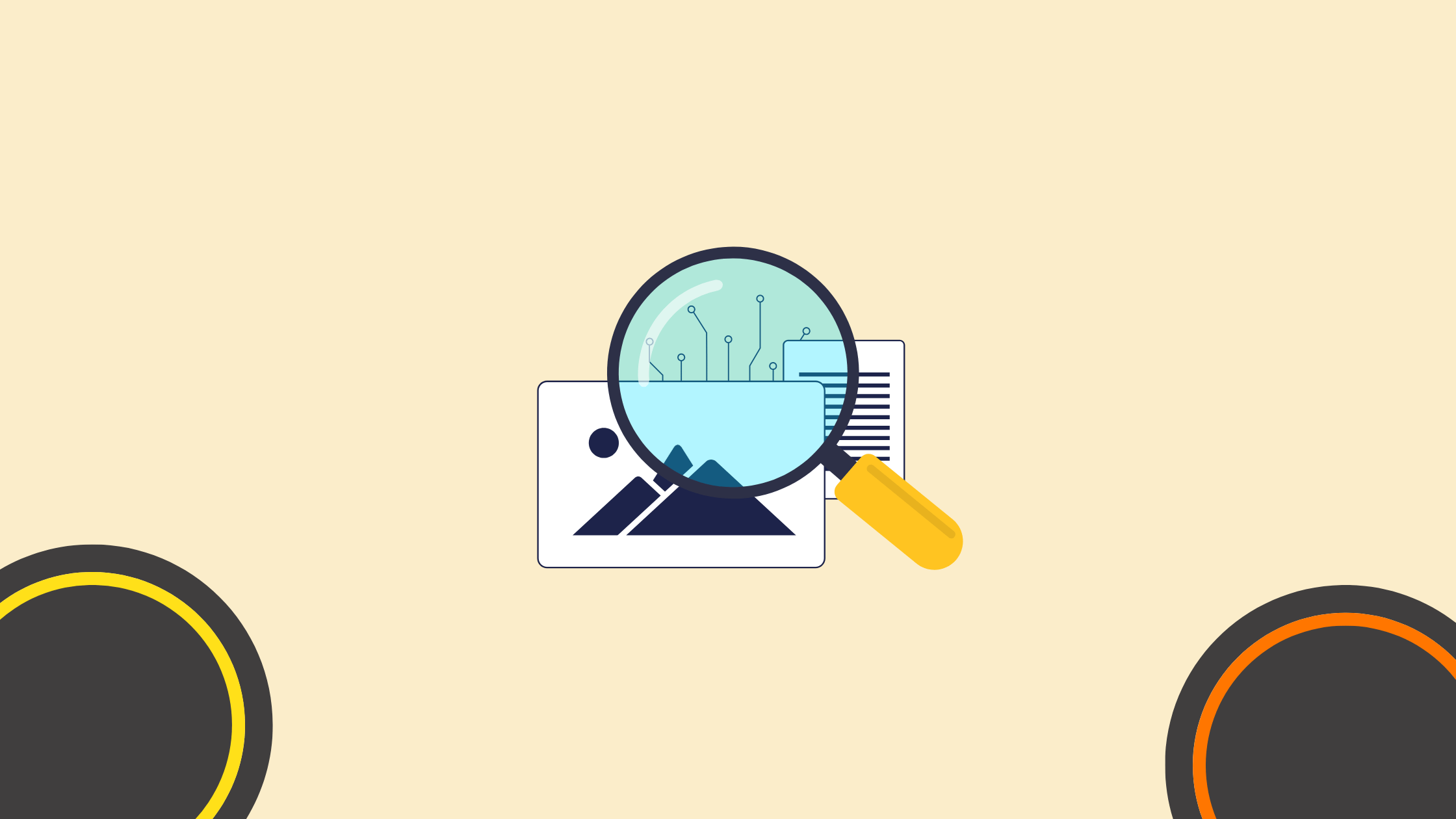
To address these concerns, researchers and developers have been working on AI detector —sophisticated algorithms designed to detect generated or manipulated content. These detectors leverage various techniques, including machine learning, computer vision, natural language processing, and statistical analysis, to identify anomalies indicative of generated content.
For instance, in the case of deepfake detection (a specific application of generative models for creating manipulated videos), AI detectors analyze subtle artifacts or inconsistencies that are not present in genuine videos. These could include discrepancies in facial expressions, blurring around the edges of manipulated objects, or inconsistencies in lighting and shadows.
Similarly, text-based AI detectors scrutinize linguistic patterns, semantic coherence, and stylistic anomalies to identify generated text that deviates from typical human writing. By leveraging large datasets of both genuine and synthetic content, these detectors can learn to distinguish between the two with high accuracy.
Challenges and Future Directions
Despite significant progress, detecting generated content remains a challenging task, primarily due to the rapid advancements in generative model technology. As generative models become more sophisticated and capable of producing increasingly realistic outputs, AI detectors must continually evolve to keep pace.
Moreover, there is a constant cat-and-mouse game between creators of generative models and developers of AI detectors. Techniques used to fool detectors are evolving alongside detection methods, leading to an ongoing arms race in AI research.
Looking ahead, interdisciplinary collaboration between experts in AI, cybersecurity, psychology, and ethics will be crucial in developing robust detection mechanisms and establishing ethical guidelines for the responsible use of generative models.
Conclusion
Generative models represent a remarkable achievement in AI research, offering unprecedented capabilities in data generation across various domains. However, their potential for misuse underscores the importance of developing effective AI detectors to safeguard against malicious activities.
As generative model technology continues to advance, so too must our efforts to detect and mitigate its negative impacts. By leveraging the power of AI to both create and defend against synthetic content, we can foster a safer and more trustworthy digital environment for all.
Master the Art of Video Marketing
AI-Powered Tools to Ideate, Optimize, and Amplify!
- Spark Creativity: Unleash the most effective video ideas, scripts, and engaging hooks with our AI Generators.
- Optimize Instantly: Elevate your YouTube presence by optimizing video Titles, Descriptions, and Tags in seconds.
- Amplify Your Reach: Effortlessly craft social media, email, and ad copy to maximize your video’s impact.