AI-based Image Restoration
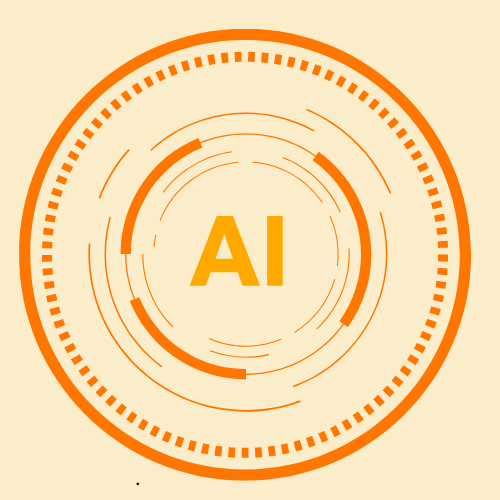
The digital age has ushered in an era of ubiquitous imagery. From medical scans to satellite photographs and personal snapshots, images play a crucial role in various scientific endeavors, historical preservation, and everyday communication. However, the quality of these images can often deteriorate due to various factors like noise introduced during acquisition, compression artifacts, or physical damage. Image restoration, the process of recovering a degraded image by removing distortions and enhancing its quality, becomes vital in such scenarios.
Saiwa as a leading company provides image restoration services. By incorporating Saiwa’s inpainting and denoising services, users can achieve high-quality image restoration, improving clarity, detail, and overall visual appeal. These services are essential for maintaining the integrity of images used in scientific research, historical preservation, and everyday applications, ensuring that degraded images are effectively restored to their optimal quality.
This article explores AI-based image restoration, detailing the fundamental principles and various applications of this technology. It is structured into several key sections: the fundamentals of image degradation, applications of AI in image restoration, specific AI techniques like image denoising AI and Image inpainting, integrated approaches, data acquisition and preprocessing, and concludes with the transformative impact of AI in this field.
Chapters
Fundamentals of Image Restoration
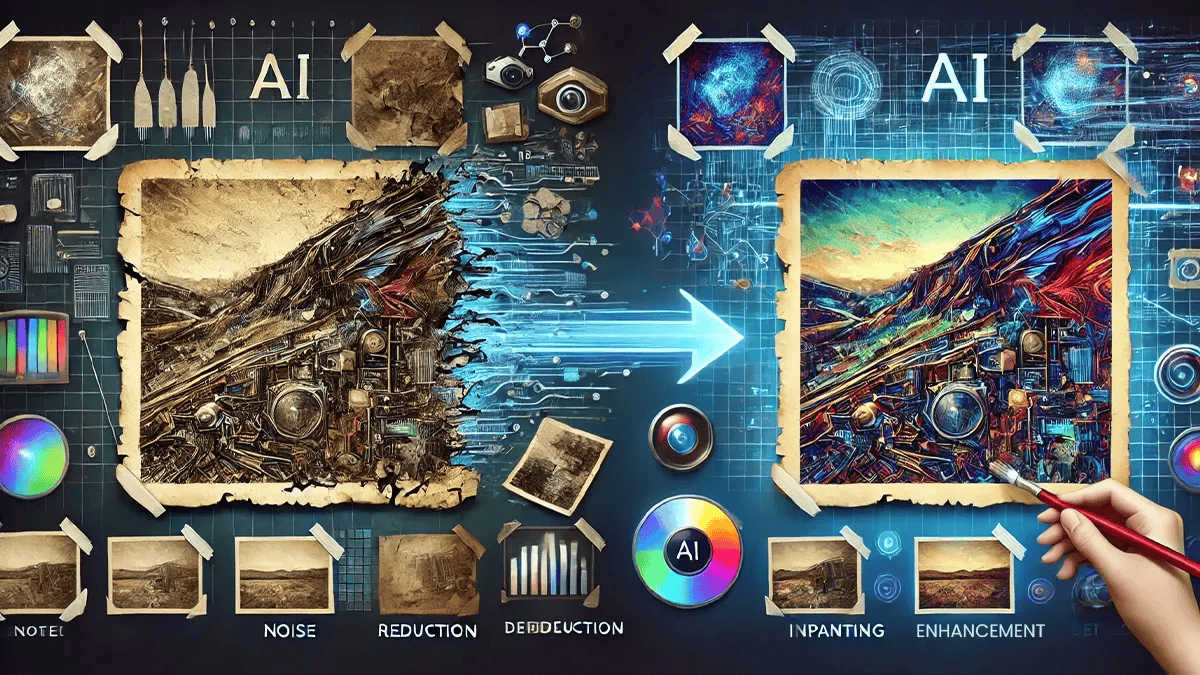
Image restoration aims to recover a high-fidelity version of a degraded image by addressing various types of distortions;
- Noise: Random variations in pixel intensity that can be introduced during image capture (e.g., sensor noise) or transmission (e.g., channel noise).
- Blur: Loss of sharpness caused by camera shake, motion blur, or out-of-focus effects.
- Compression artifacts: Distortions introduced during image compression to reduce file size.
- Physical damage: Scratches, tears, or other physical damage to the image source (e.g., old photographs).
Applications of AI-based Image Restoration
The emergence of artificial intelligence (AI) has revolutionized image restoration, offering powerful tools to address diverse degradation challenges. Here are some key application areas:
1- Medical imaging
Restoring medical images like X-rays, MRI scans, or CT scans is crucial for accurate diagnosis and treatment planning. AI can improve image quality by removing noise, enhancing resolution, and reducing artifacts.
2- Satellite and aerial imagery
Satellite and aerial images are vital for applications like environmental monitoring, land-use mapping, and disaster response. AI-based restoration can improve the quality of these images by removing noise.
3- Photography and image editing
AI tools can significantly enhance the user experience in photography and image editing. Image restoration capabilities can help photographers recover noisy or blurry images captured in low-light conditions.
AI and Deep Learning in Image Restoration
How AI enhances image restoration processes:
- Learning from data: Unlike traditional methods, AI learns the restoration process directly from data, achieving superior performance on complex degradation scenarios.
- Adaptability: AI models can be trained on diverse types of degradation, making them adaptable to various image restoration tasks.
- Automation: AI-based image restoration can be automated, reducing the need for manual intervention and expertise in traditional restoration methods.
Overview of neural networks, especially Convolutional Neural Networks (CNNs)
- Neural networks: These are loosely inspired by the structure and function of the human brain and consist of interconnected layers of artificial neurons.
- Convolutional Neural Networks (CNNs): A specific type of neural network architecture particularly well-suited for image processing tasks.
Image Denoising
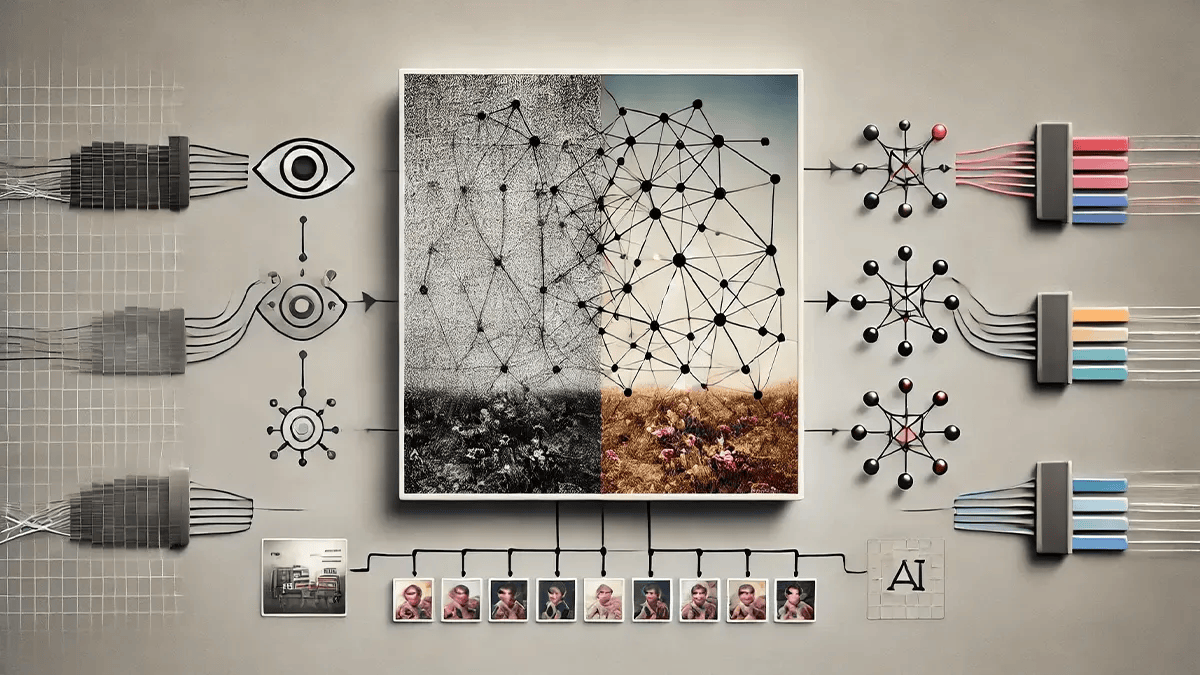
Noise is a common form of image degradation that can significantly impact image quality. AI offers powerful tools for image denoising ai:
Understanding Image Noise
Additive noise, like Gaussian noise, adds random variations to pixel intensities. Multiplicative noise, like salt-and-pepper noise, replaces individual pixels with extreme intensity values (black or white). Using advanced algorithms, Saiwa platform effectively reduces noise while preserving the essential details and textures of the original image.
AI Techniques for Image Denoising
- Autoencoders: In image denoising, an autoencoder is trained to reconstruct the clean image from the noisy input, effectively removing noise in the process.
- Generative Adversarial Networks (GANs): These involve two competing neural networks: a generator and a discriminator.
- Applications: Image denoising using AI has a wide range of applications, including:
-
- Medical imaging: Removing noise from medical images can enhance diagnostic accuracy by improving visualization of subtle details.
- Astronomy: AI can denoise astronomical images, revealing faint features in deep-space observations.
- Low-light photography: AI denoising can significantly improve the quality of photographs taken in low-light conditions, where noise is often a significant issue.
Image Inpainting
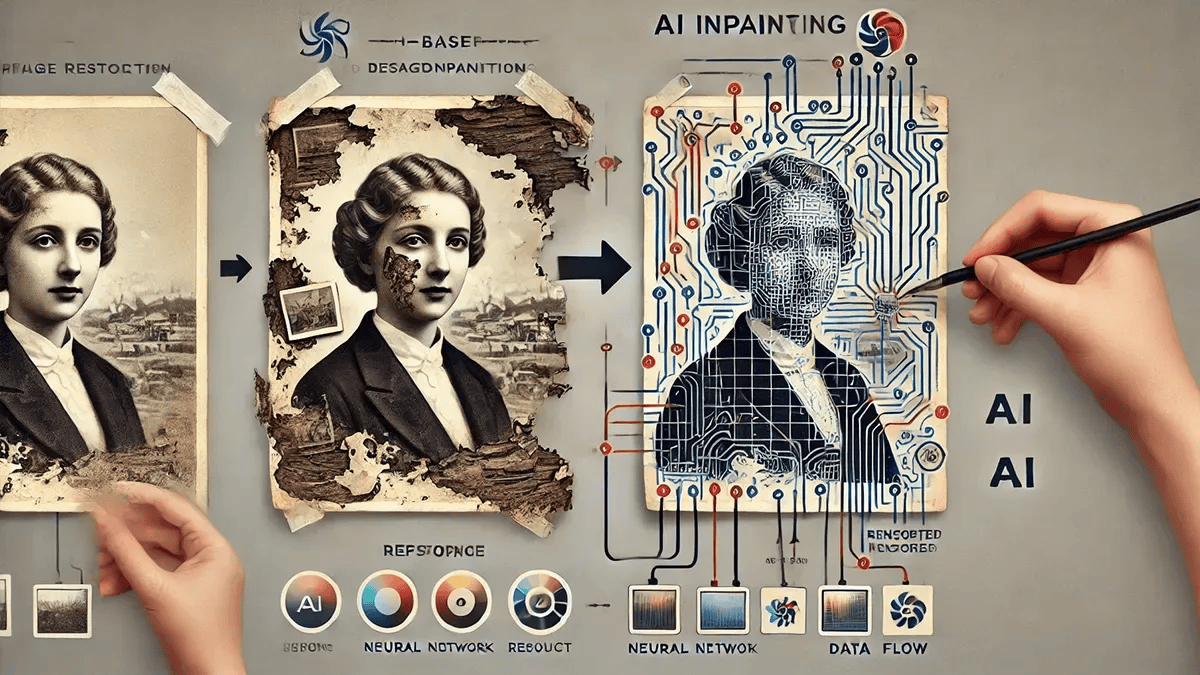
Understanding Image Inpainting
Image inpainting involves analyzing the surrounding intact areas of the image to infer and reconstruct the missing content. This requires the AI model to understand the context and spatial relationships within the image. Saiwa’s inpainting service is designed to restore missing or corrupted regions of images. This technique involves reconstructing the damaged parts of an image using information from the surrounding areas, ensuring that the restored sections blend seamlessly with the rest of the image.
AI Techniques for Image Inpainting
- Patch-based methods: These methods break down the image into small patches and leverage the redundancy within the image to find similar patches that can be used to fill in the missing regions.
- Generative models:The model learns to generate plausible image content that fills the missing area, similar to how Flux1 enhances image processing capabilities.
Applications
- Art restoration: Damaged paintings or historical photographs can be restored by filling in missing or degraded areas.
- Movie and video editing: Removing unwanted objects or blemishes from videos can be achieved.
- Medical imaging: Inpainting can be used to address artifacts or missing data in medical scans, improving image quality for diagnostic purposes.
Integrated Approaches
While image denoising and inpainting can be addressed as separate tasks, there is a growing trend towards integrated approaches:
Combining image denoising and inpainting in a unified framework
Recent research explores combining denoising and inpainting techniques into a single AI model.
Example frameworks and algorithms that handle both denoising and inpainting
- Deep Image Prior (DIP): This framework leverages a deep neural network to learn a prior distribution of natural images. By incorporating this prior into the restoration process, DIP can effectively handle both noise removal and content inpainting.
- Partial Convolutional Layers (PCL): This approach utilizes a CNN architecture with specifically designed convolutional layers that can handle both complete and missing regions of the image.
Data Acquisition and Preprocessing
Importance of high-quality data for training AI models
High-quality data, consisting of pairs of degraded and corresponding high-quality images, is essential for the model to learn effective restoration strategies.
Methods for acquiring training data
- Simulated degradation: Realistic degradation models can be used to artificially degrade high-quality images, creating a vast dataset for training purposes.
- Crowdsourcing: Online platforms can be used to collect user-generated content, where users provide both degraded and restored versions of images.
Conclusion
AI-based image restoration is revolutionizing the way we recover and enhance degraded images. By leveraging deep learning techniques, AI models can remove noise, fill in missing content, and achieve remarkable restoration results that were previously difficult or impossible to attain with traditional methods.
Author Bio
Thomas Lore is a 28-year old writer. As a creative and diligent freelance blogger, he is always seeking new ways to improve himself.
Other Interesting Articles
Master the Art of Video Marketing
AI-Powered Tools to Ideate, Optimize, and Amplify!
- Spark Creativity: Unleash the most effective video ideas, scripts, and engaging hooks with our AI Generators.
- Optimize Instantly: Elevate your YouTube presence by optimizing video Titles, Descriptions, and Tags in seconds.
- Amplify Your Reach: Effortlessly craft social media, email, and ad copy to maximize your video’s impact.