Exploring Sentiment Analysis with Generative AI
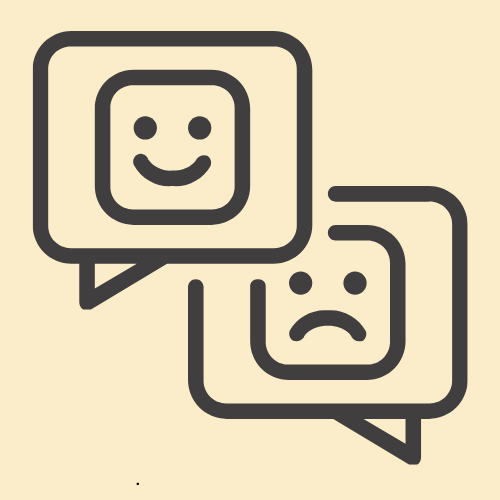
We have come to the stage where machines can now interpret text to identify hidden emotions and perceptions. Sentiment analysis harnesses machine learning, artificial intelligence, and computational linguistics to determine the emotional tone behind a context.
Companies conduct sentiment analysis or opinion mining to gather insights into customer behavior and use them to improve customer experience. Generative AI can also help with sentiment analysis by creating text samples with specific emotional characteristics.
Read ahead as we take a detailed overview of how GenAI is helpful for sentiment analysis.
Chapters
Reasons to Use Generative AI for Sentiment Analysis
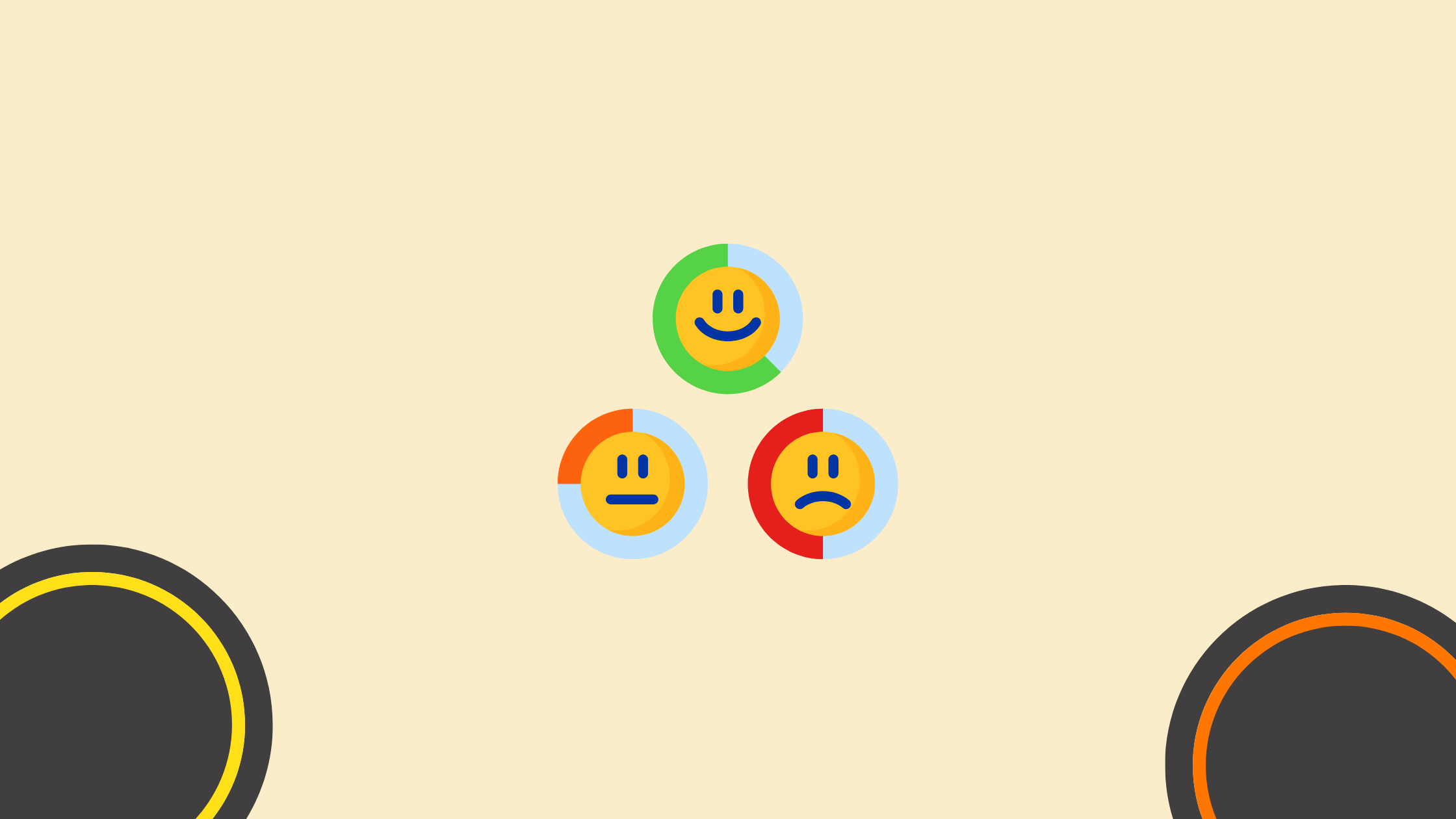
Generative AI is hailed as a game changer for sentiment analysis, offering some unique advantages over other methods used for the same purpose.
Better Accuracy through Training
AI models are trainable. Using specific and vast datasets, generative models can be trained, allowing them to pick up subtle linguistic concepts like irony, sarcasm, slang, etc. As a result, generative AI models can provide higher accuracy with sentiment analysis.
In addition to training on multiple emotions, we can train them in different languages. Companies can run sentiment analysis with fewer limitations and better understand their customers.
Higher Efficiency and Easy to Scale
Generative AI models analyze large volumes of text data in real-time, enabling quicker identification of trends and shifts in sentiment. This is highly useful for monitoring sentiment on social media and improving customer service.
In terms of scalability, the GenAI models can be quickly scaled according to the requirements. They can also be customized to fit the needs of any industry and sector.
While generative AI is useful for text classification, exercising caution is necessary due to a few limitations. Like any AI model, generative models can also inherit biases through training data and deliver skewed results.
Moreover, the training and computational costs of training are huge. However, with Shaip, you can optimize the NLP sentiment analysis cost, and we use verified and tested data for training purposes to build accurate models.
Capabilities of Generative AI for Sentiment Analysis
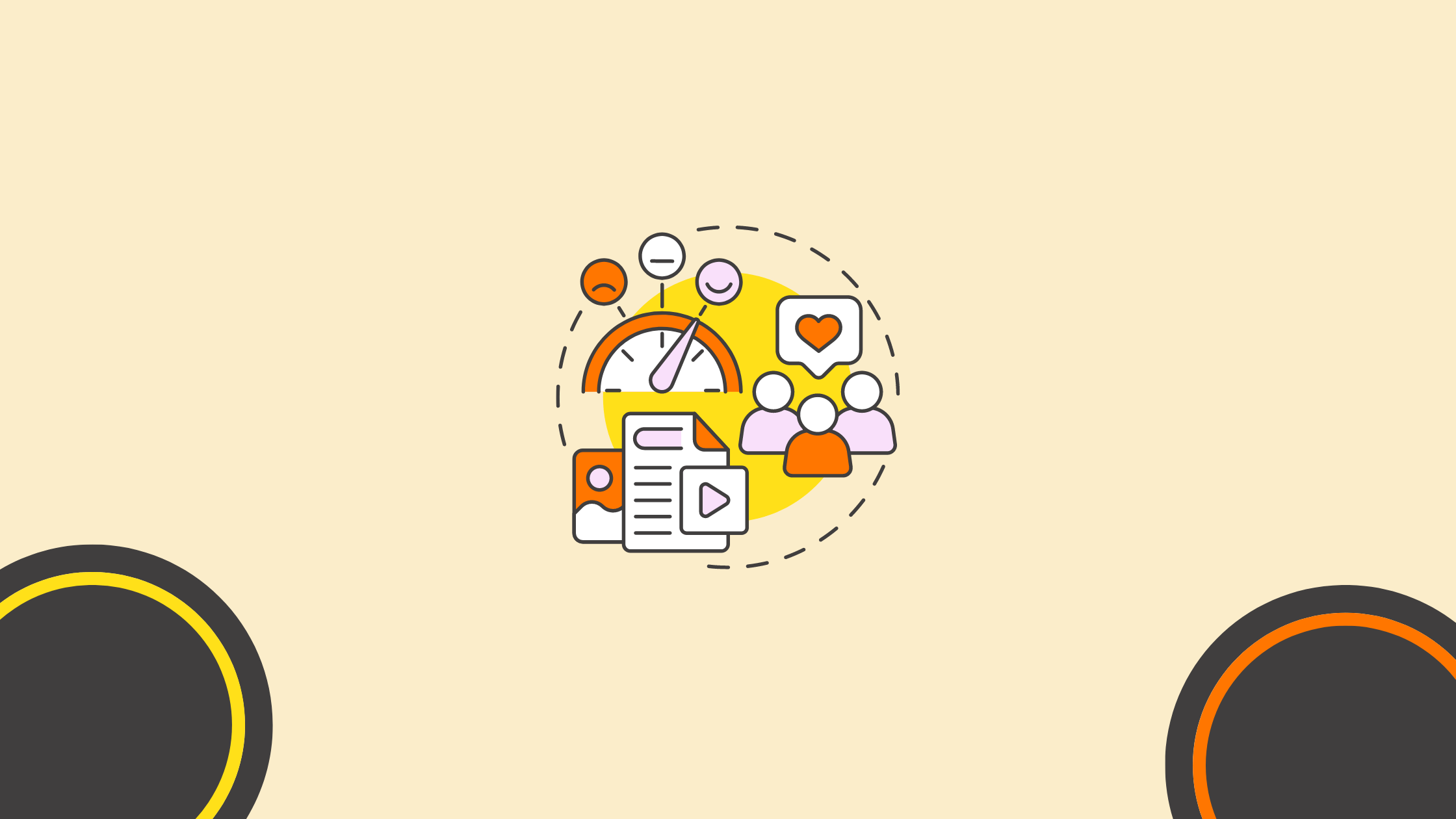
Generative AI models take the processing and analysis one step further and are useful in each stage of sentiment analysis. These are;
- Preprocessing Data: GenAI models can be used to create artificial or synthetic data for pre-training models. They can also be used to clean AI models and improve their efficiency.
- Context Understanding: Generative AI contextual analysis goes one step ahead from pattern matching to include capturing in-depth cues and nuances, leading to higher accuracy.
- Sentiment Classification: We can train the advanced AI models to achieve higher accuracy through sentiment classification data. This means that instead of classifying data as positive, negative, or neutral, the GenAI models can identify text samples with a specific emotion.
GenAI models can generate realistic training data to improve sentiment analysis through these capabilities. It can capture complex information, offering more accurate opinion mining and personalizing the analytical exercise according to your requirements.
Use Cases of Generative AI for Sentiment Analysis
GenAI can be used for sentiment analysis in different ways and enable users to improve their processes while optimizing operations. Here are a few applications of generative AI to help you understand its role.
Social Media Monitoring: GenAI models, after analyzing vast amounts of social media data, can detect brand mentions and note their sentiments, giving you a general overview of public opinion.
Engaging with positive and negative feedback on social media in real time proves effective for brand reputation management.
Customer Experience Enhancement: Through text classification, these models can analyze customer reviews, emails, tickets, and chatbot conversations to identify the emotional drivers behind every sentiment.
With its natural language processing capabilities, the GenAI models can also generate personalized responses to customer inquiries and concerns. Ultimately, it can also identify areas of improvement and sentiment trends to optimize the customer experience journey. Just make sure to use reinforcement learning from AI feedback (RLAIF) when working with these models to continuously improve the chatbot’s performance and ensure it delivers accurate and helpful information.
Product Development Through Market Research: Another aspect of analyzing customer interactions in reviews and other user-generated content is knowing their preferences. This information is crucial to gauging customer’s product perception and strategizing the product development phase.
Based on the interests and preferences generated by the generative AI models, you can build customized marketing campaigns.
Personalized Content Creation: GenAI’s NLP capabilities can be utilized to generate personalized content for product descriptions, ads, social media posts, etc. Moreover, you can generate content that evokes specific emotions in the audiences according to their preferences.
Similarly, it can be used to personalize feeds, make music recommendations, and suggest movies based on individual interests.
For these applications, two types of generative models are used:
- Generative Adversarial Networks (GAN): These are models with a generator and a discriminator. Where the generator is responsible for producing synthetic text, the discriminator distinguishes between actual and generated text.
- Variational Autoencoders: VAEs are probabilistic generative models with neural network components, encoder, and decoder. In GenAI for sentiment analysis, VAEs capture the sentiment distribution of a given data set and generate new text with specific emotional characteristics.
Benefit from Efficient Sentiment Analysis
Sentiment analysis is a crucial aspect of product development, product improvement, and marketing. Businesses looking to harness generative AI for sentiment analysis will benefit from the model’s accuracy, scalability, and flexibility.
FAQ
What is sentiment analysis?
Sentiment analysis is the process of determining the emotional tone behind a series of words, used to understand the attitudes, opinions, and emotions expressed in textual data.
How does generative AI enhance sentiment analysis?
Generative AI improves sentiment analysis by generating more accurate predictions, understanding context, handling nuances in language, and providing more detailed insights into emotions and sentiments.
What are the applications of sentiment analysis with generative AI?
Applications include customer feedback analysis, social media monitoring, brand reputation management, market research, and enhancing customer service through sentiment-aware interactions.
How does generative AI work in sentiment analysis?
Generative AI uses models like GPT-4 to analyze text, generate possible interpretations of sentiment, and provide contextual understanding, which enhances the accuracy and depth of sentiment analysis.
What are the benefits of using generative AI for sentiment analysis?
Benefits include improved accuracy, the ability to analyze large volumes of data, better context understanding, real-time analysis, and the ability to generate actionable insights.
What types of data can be analyzed using sentiment analysis with generative AI?
Types of data include social media posts, customer reviews, survey responses, emails, forums, blogs, and any other text-based content.
How accurate is sentiment analysis with generative AI?
Accuracy depends on the quality of the data and the AI model used, but generative AI generally provides higher accuracy than traditional methods due to its advanced natural language processing capabilities.
What are the challenges of using generative AI for sentiment analysis?
Challenges include handling sarcasm and irony, understanding context-specific sentiments, managing diverse language nuances, and ensuring data privacy and security.
How can businesses implement sentiment analysis with generative AI?
Businesses can implement this by integrating generative AI models with their data analytics tools, using APIs provided by AI platforms, and leveraging custom AI solutions tailored to their specific needs.
What are some popular tools for sentiment analysis with generative AI?
Popular tools include OpenAI’s GPT models, IBM Watson Natural Language Understanding, Google Cloud Natural Language API, and Microsoft Azure Text Analytics.
How does generative AI handle multilingual sentiment analysis?
Generative AI can be trained on multilingual datasets, allowing it to understand and analyze sentiments expressed in multiple languages, making it useful for global businesses.
What is the role of training data in sentiment analysis with generative AI?
Training data is crucial as it helps the AI model learn and understand language patterns, emotions, and context, which enhances its ability to accurately analyze sentiments.
Can generative AI identify sarcasm in sentiment analysis?
Generative AI can identify sarcasm to some extent by recognizing context and language cues, but it is still a challenging area requiring continuous improvement.
How can sentiment analysis with generative AI improve customer service?
It can analyze customer interactions to identify negative sentiments, allowing businesses to address issues proactively and tailor responses to improve customer satisfaction.
What is the impact of sentiment analysis with generative AI on marketing strategies?
It provides insights into customer emotions and preferences, helping businesses craft targeted marketing campaigns, improve product offerings, and enhance overall customer engagement.
How do you measure the success of sentiment analysis with generative AI?
Success can be measured by the accuracy of sentiment predictions, the ability to generate actionable insights, improvements in customer satisfaction, and the effectiveness of subsequent business strategies.
What are the ethical considerations when using generative AI for sentiment analysis?
Ethical considerations include ensuring data privacy, avoiding biases in AI models, obtaining consent for data use, and being transparent about AI’s role in sentiment analysis.
How does generative AI compare to traditional sentiment analysis methods?
Generative AI offers more advanced language understanding, better context recognition, and higher accuracy compared to traditional methods, which rely on predefined rules and simpler algorithms.
What industries can benefit from sentiment analysis with generative AI?
Industries such as retail, finance, healthcare, entertainment, hospitality, and any sector that relies on customer feedback and public opinion can benefit from this technology.
What is the future of sentiment analysis with generative AI?
The future includes more sophisticated models with higher accuracy, real-time sentiment tracking, deeper contextual understanding, and broader applications across various industries.
Other interesting reads
The Psychology Behind Choosing The Right Color For Your App Designing [...]
Get Your Web Site Up and Running: Dedicated Server Hosting Is the [...]
Top 6 Proven Tips to Humanize AI Content Today [...]
Best Gardening Apps to Cultivate Your Dream Garden in 2025 [...]
Top 10 Best Email Verification Tools in 2025 [...]
How AI Improves Online Retail Consumer Experience [...]
Author Bio
I’m Erika Balla, a Hungarian from Romania with a passion for both graphic design and content writing. Following the completion of my studies in graphic design, I discovered a second passion in content writing, particularly in crafting well-researched, technical articles. I derive joy from dedicating hours to reading magazines and collecting materials that inspire the creation of my articles. What sets me apart is my love for precision and aesthetics. I am committed to delivering high-quality content that not only educates but also engages readers with its visual appeal. I bring a unique perspective to my writing, actively immersing myself in this field to produce articles that illuminate complex concepts and present them in a clear and accessible manner.
Master the Art of Video Marketing
AI-Powered Tools to Ideate, Optimize, and Amplify!
- Spark Creativity: Unleash the most effective video ideas, scripts, and engaging hooks with our AI Generators.
- Optimize Instantly: Elevate your YouTube presence by optimizing video Titles, Descriptions, and Tags in seconds.
- Amplify Your Reach: Effortlessly craft social media, email, and ad copy to maximize your video’s impact.