Practical Applications of Artificial Intelligence in Data Science
In the ever-evolving landscape of data science, the incorporation of Artificial Intelligence (AI) has ushered in a transformative era, bringing forth practical applications that redefine how organizations harness the power of their data. This dynamic synergy between AI and data science is not just theoretical but has tangible implications across various domains.
Let’s delve into some practical applications where AI is making significant contributions to the field of data science. Understanding and mastering these applications is essential for professionals seeking to advance their careers, making specialized training such as Data Scientist Certification a crucial investment in staying at the forefront of this rapidly evolving field.
Chapters
Predictive Analytics
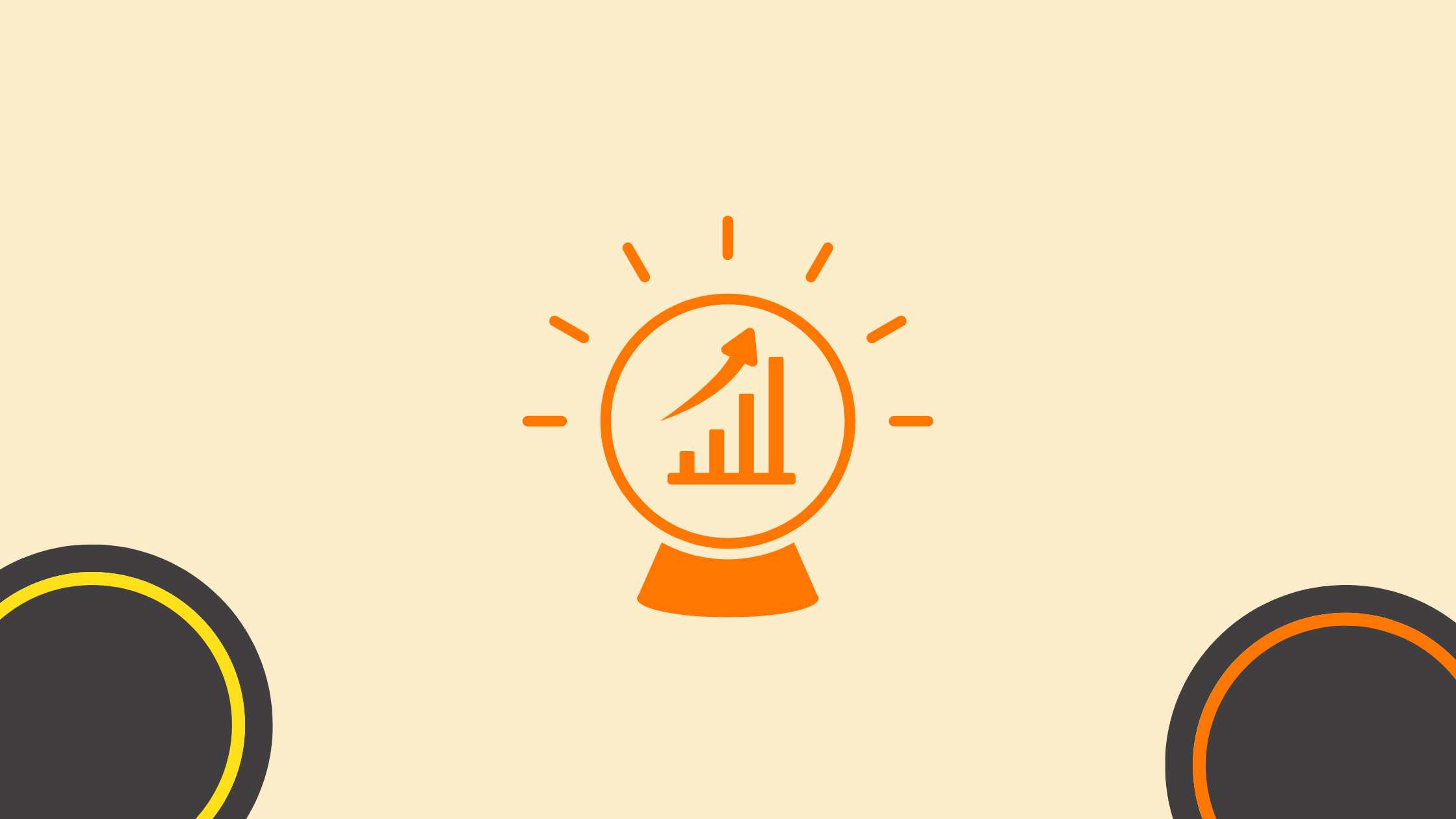
Predictive analytics powered by AI algorithms is a cornerstone of data science applications. In finance, these algorithms analyze historical stock data, enabling the prediction of future stock prices. Healthcare leverages predictive analytics for disease prognosis by examining patient data, while marketing utilizes it to forecast customer behavior. The ability to identify patterns within extensive datasets allows organizations to make informed decisions based on predictive insights, optimizing strategies, and resource allocation.
Natural Language Processing (NLP)
Natural Language Processing (NLP), nestled within the broader domain of Artificial Intelligence (AI), serves as a key enabler for machines to understand and interpret human language. In the realm of data science, NLP stands out as a powerful tool, employed to analyze textual data and extract valuable insights from unstructured content. Let’s delve into the multifaceted applications of NLP, showcasing its versatility in enhancing information extraction and understanding across various industries.
One prominent application of NLP is sentiment analysis, a process where machines assess and comprehend the opinions expressed in textual content. Whether it’s user reviews, social media posts, or customer feedback, sentiment analysis enables organizations to gauge the emotional tone and sentiment behind the text. This invaluable information empowers businesses to adapt their strategies based on public perception, contributing to more informed decision-making in marketing, product development, and customer engagement.
Language translation is another area where NLP demonstrates its prowess. Breaking down language barriers, NLP facilitates the automatic translation of text from one language to another. This capability is particularly significant in our interconnected global landscape, fostering effective communication and collaboration across diverse linguistic contexts. Industries engaged in international business, diplomacy, and content localization leverage NLP for seamless cross-cultural interactions.
Chatbots represent a tangible manifestation of NLP in action, where machines engage in natural language conversations with users. These AI-driven virtual assistants comprehend user queries, respond appropriately, and simulate human-like interactions. In customer support, chatbots can handle routine inquiries, provide information, and guide users through processes, enhancing efficiency and freeing up human agents for more complex tasks. This application of NLP contributes to improved customer experiences and operational effectiveness.
NLP’s ability to process large volumes of text data is a game-changer in industries dealing with extensive textual information. In healthcare, for example, NLP can extract relevant information from medical records, aiding in clinical decision-making and research. In the legal sector, NLP assists in the analysis of legal documents, improving document review processes and facilitating legal research. Similarly, in finance, NLP enhances the extraction of insights from financial reports and market analyses.
Image and Video Analysis
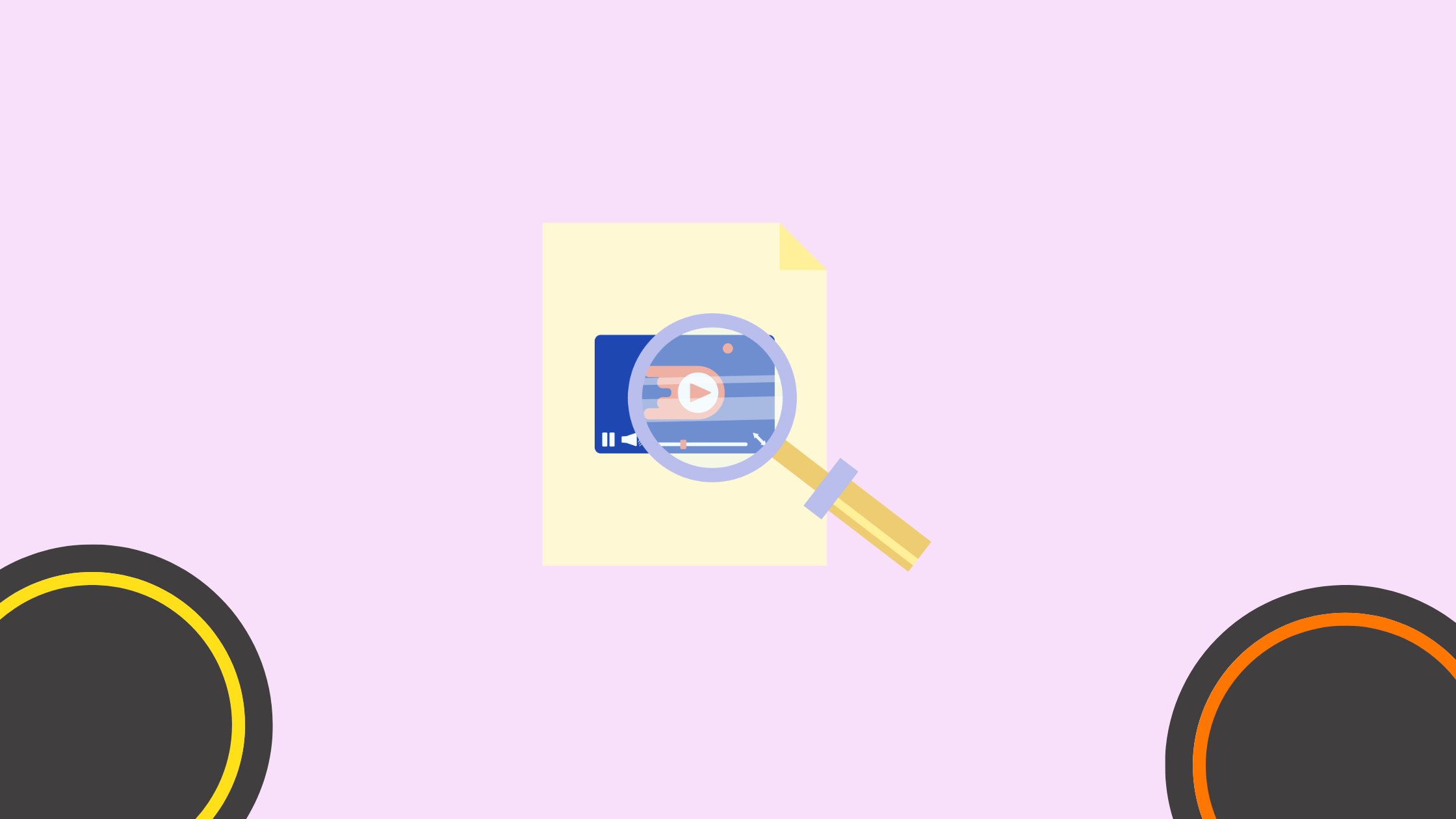
The integration of AI and data science has revolutionized image and video analysis. Deep learning models excel in facial recognition, object detection, and image classification. Security benefits from facial recognition, healthcare utilizes image analysis for diagnostics, and retail enhances processes like identity verification and product recognition. The precision and efficiency brought by AI-driven image and video analysis transform industries, improving security, healthcare, and customer experiences.
Recommendation Systems
AI-driven recommendation systems have become integral to online platforms, leveraging data science to provide personalized suggestions. These systems analyze user behavior and preferences to enhance user experience. From content recommendations on streaming services to product suggestions in e-commerce, recommendation systems improve engagement and satisfaction. By understanding user preferences, organizations can tailor offerings, increasing customer loyalty and driving revenue.
Anomaly Detection
Anomaly detection, powered by AI algorithms, is pivotal in identifying irregularities within datasets. In finance, it helps detect fraudulent transactions, safeguarding financial systems. In manufacturing, anomaly detection pinpoints defects, improving product quality. With tools like QuickBooks data integration, AI algorithms can seamlessly analyze financial data, learn normal patterns, and efficiently flag deviations for investigation. Anomaly detection enhances risk management, security, and quality control, contributing to the overall efficiency and integrity of processes.
Automated Machine Learning (AutoML)
Automated Machine Learning (AutoML) represents a breakthrough in streamlining the machine learning process. AI automates tasks like feature engineering and model selection, democratizing machine learning. By enabling efficient model building without extensive manual intervention, AutoML accelerates the development of machine-learning applications. This accessibility empowers data scientists and analysts, fostering innovation and driving the adoption of machine learning in various domains.
Clustering and Segmentation
AI algorithms contribute to clustering and segmentation in data science by grouping similar data points. In customer segmentation for targeted marketing, organizations can tailor strategies based on shared characteristics. Clustering aids in organizing large datasets for meaningful analysis, enhancing decision-making. In information retrieval, grouping similar documents streamlines data access and management. The applications of clustering and segmentation span industries, optimizing processes and insights derived from data.
Time Series Forecasting
AI-powered time series forecasting is instrumental in predicting future trends based on historical patterns. In finance, these models analyze past market trends, aiding in resource allocation and risk management. Weather forecasting benefits from AI-powered time series analysis, improving prediction accuracy. The ability to anticipate future trends enhances strategic planning in sales and other industries. AI-driven time series forecasting contributes to data-driven decision-making, optimizing outcomes in diverse fields.
Conclusion
The practical applications of AI in data science are transformative tools with real-world impact. The synergy between AI and data science continues to evolve, necessitating continuous learning for professionals in the field. Investment in specialized “Data Science and AI Training” through a Data Science online course is crucial for staying at the forefront of this rapidly evolving landscape, ensuring organizations can leverage the full potential of AI in data science applications. The applications explored here highlight the diverse and profound ways in which AI is reshaping decision-making, insights generation, and overall efficiency across industries.
FAQ
What is AI in data science?
AI in data science refers to the application of artificial intelligence techniques and algorithms to analyze, interpret, and leverage data to make predictions, identify patterns, and inform decision-making.
How does AI enhance data science?
AI enhances data science by automating data processing tasks, improving the accuracy of predictive models, enabling real-time data analysis, and uncovering insights that may be difficult to detect using traditional methods.
What are the benefits of using AI in data science?
Benefits include increased efficiency, more accurate predictions, the ability to process large datasets quickly, enhanced data visualization, and the automation of routine tasks.
What are the common AI techniques used in data science?
Common AI techniques include machine learning, deep learning, natural language processing (NLP), computer vision, and neural networks.
How does machine learning contribute to data science?
Machine learning algorithms analyze historical data to identify patterns and build models that can predict future outcomes, continuously improving their accuracy over time.
What is the role of deep learning in data science?
Deep learning, a subset of machine learning, uses neural networks with multiple layers to process and analyze complex data, such as images and speech, to generate more nuanced insights.
How is natural language processing (NLP) used in data science?
NLP enables the analysis and interpretation of human language data, facilitating tasks such as sentiment analysis, text classification, and the extraction of insights from unstructured text data.
What industries benefit from AI in data science?
Industries such as healthcare, finance, retail, manufacturing, and marketing benefit from AI in data science by optimizing operations, improving customer experiences, and driving innovation.
How does AI improve predictive analytics in data science?
AI enhances predictive analytics by building more accurate and robust models that can forecast future trends and behaviors, helping businesses make data-driven decisions.
What are the challenges of integrating AI in data science?
Challenges include the need for large datasets, ensuring data quality, managing the complexity of AI models, the requirement for specialized skills, and addressing ethical and privacy concerns.
How does AI help in data preprocessing?
AI automates data preprocessing tasks such as data cleaning, normalization, and transformation, reducing the time and effort required to prepare data for analysis.
What tools are commonly used for AI in data science?
Common tools include TensorFlow, PyTorch, Scikit-learn, Keras, IBM Watson, and Apache Spark, each offering various functionalities for building and deploying AI models.
How does AI enable real-time data analysis?
AI processes data at high speeds, allowing for real-time analysis and immediate insights, which is crucial for applications like fraud detection, real-time recommendations, and dynamic pricing.
What is the difference between AI and traditional data analysis?
Traditional data analysis often relies on predefined algorithms and statistical methods, while AI leverages machine learning and other techniques to automatically identify patterns and make predictions without explicit programming.
How do AI and big data work together in data science?
AI and big data complement each other by using AI algorithms to analyze and extract valuable insights from vast and complex datasets, driving more informed decision-making.
What is the importance of data quality in AI-driven data science?
High-quality data is crucial for training accurate AI models, as poor data quality can lead to incorrect insights and predictions, undermining the reliability of the results.
How can businesses implement AI in their data science processes?
Businesses can implement AI by identifying use cases, investing in AI and data science talent, using appropriate tools and technologies, and fostering a data-driven culture within the organization.
What ethical considerations should be taken into account with AI in data science?
Ethical considerations include ensuring data privacy, preventing bias in AI models, maintaining transparency, and adhering to regulatory standards and guidelines.
How does AI handle unstructured data in data science?
AI techniques like NLP and computer vision can analyze unstructured data, such as text, images, and videos, extracting meaningful insights that structured data analysis methods might miss.
What is the future of AI in data science?
The future of AI in data science includes more advanced and interpretable models, greater integration with other technologies, increased automation, and the continued evolution of AI to address complex data challenges and drive innovation.
Master the Art of Video Marketing
AI-Powered Tools to Ideate, Optimize, and Amplify!
- Spark Creativity: Unleash the most effective video ideas, scripts, and engaging hooks with our AI Generators.
- Optimize Instantly: Elevate your YouTube presence by optimizing video Titles, Descriptions, and Tags in seconds.
- Amplify Your Reach: Effortlessly craft social media, email, and ad copy to maximize your video’s impact.